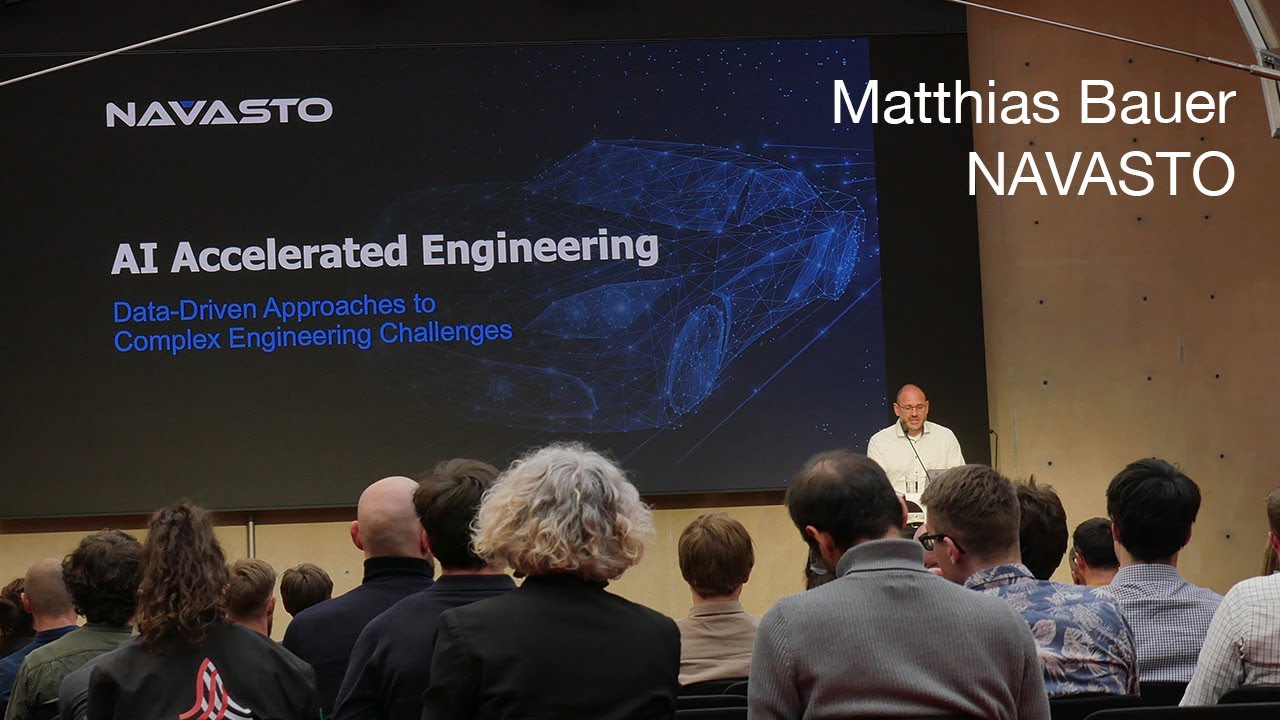
Data-Driven Engineering Solutions | An Interview by CDFAM
Explore AI’s impact on design, development processes, and multi-objective optimization with insights from Matthias Bauer on data-driven engineering solutions.
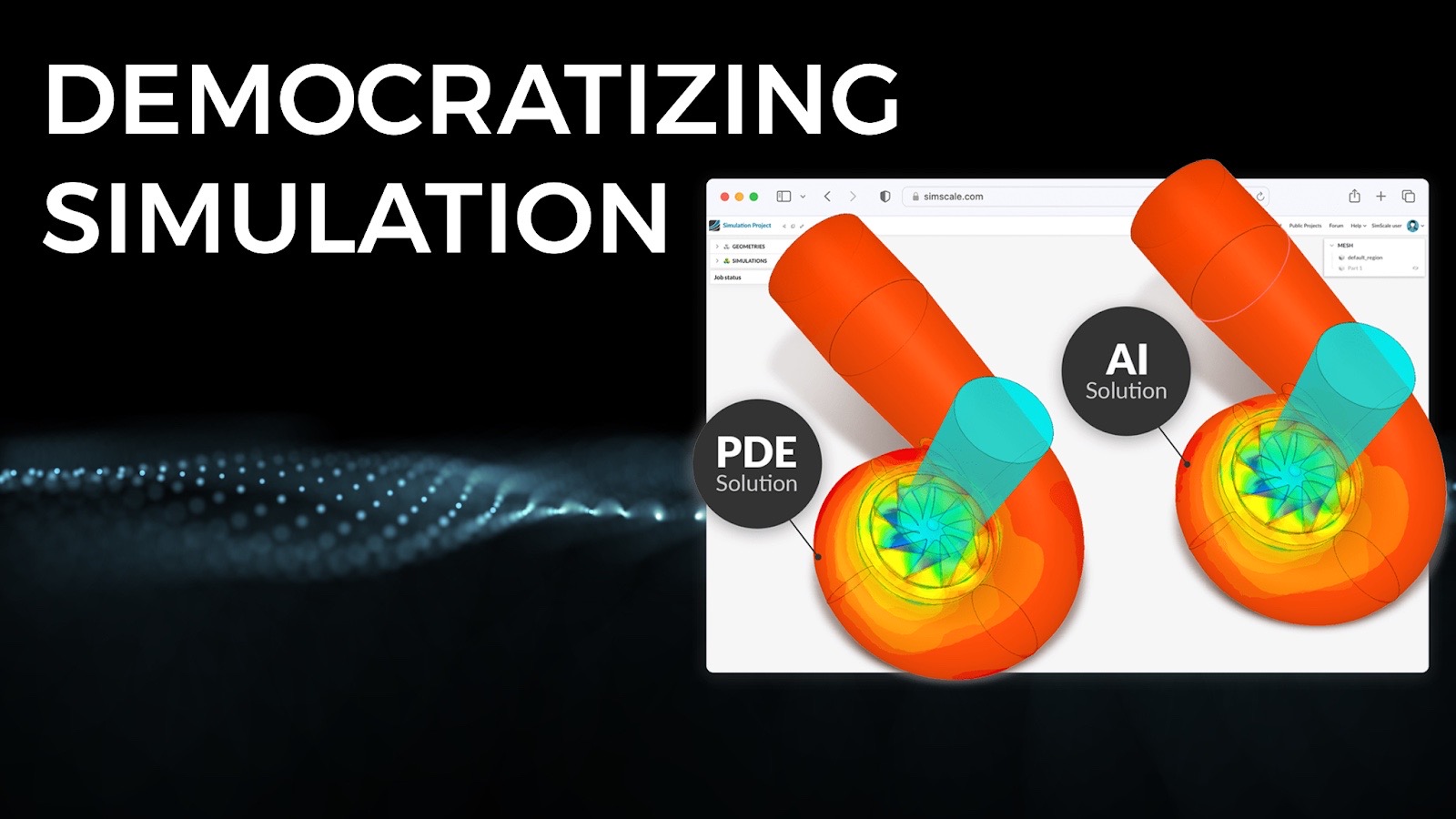
The Power of Democratizing Engineering through Left-Shifting and Simulation-Driven Decisions
Learn how the democratization of simulation allows for smarter and faster design decisions. Explore AI integration, left-shifting strategies, and how NAVASTO’s advanced solutions are transforming product development.
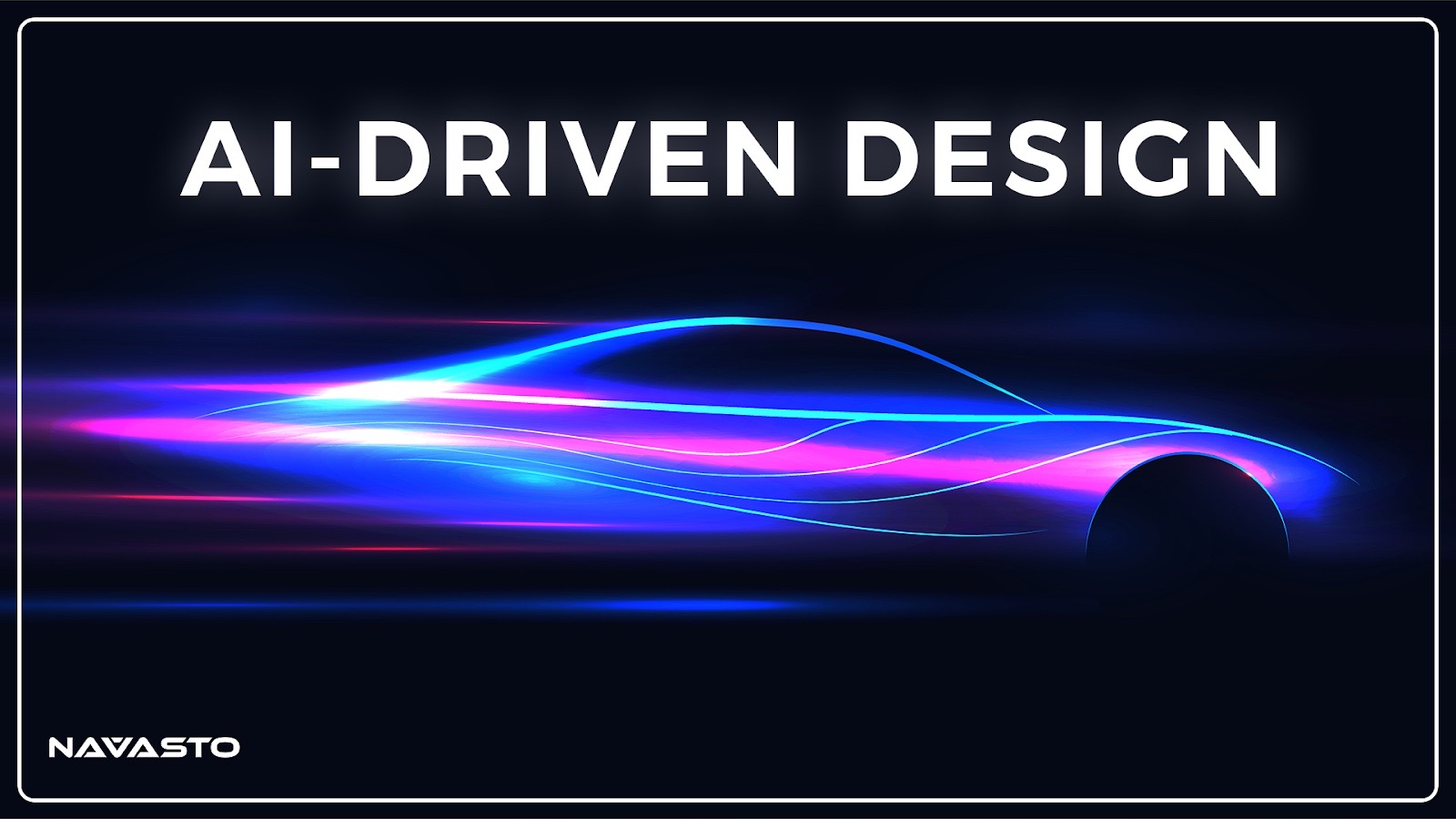
Design at the Speed of Thought: Exploring AI-Driven Automotive Design and Optimization
Explore the significant enhancements in vehicle development efficiency and design precision brought by generative design and real-time engineering.
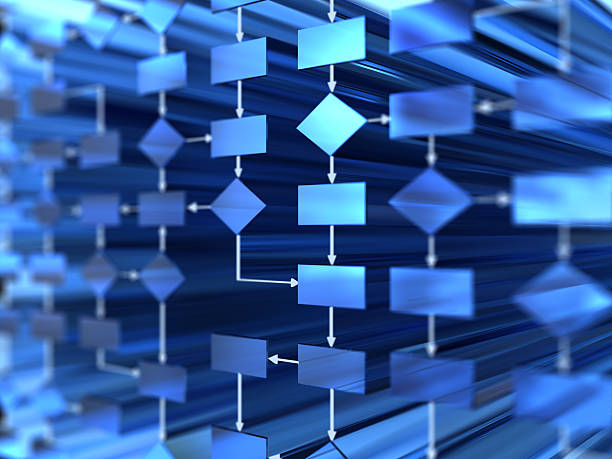
How to Optimize Your 3D Workflows With Advanced Geometry Modification and Visualization
NAVASTO’s AI-driven solutions transform engineering workflows, accelerating development cycles, reducing costs, and empowering innovation. From real-time CFD predictions to streamlined design workflows, discover how advanced geometry modification and visualization can optimize your 3D workflows for enhanced productivity and efficiency.
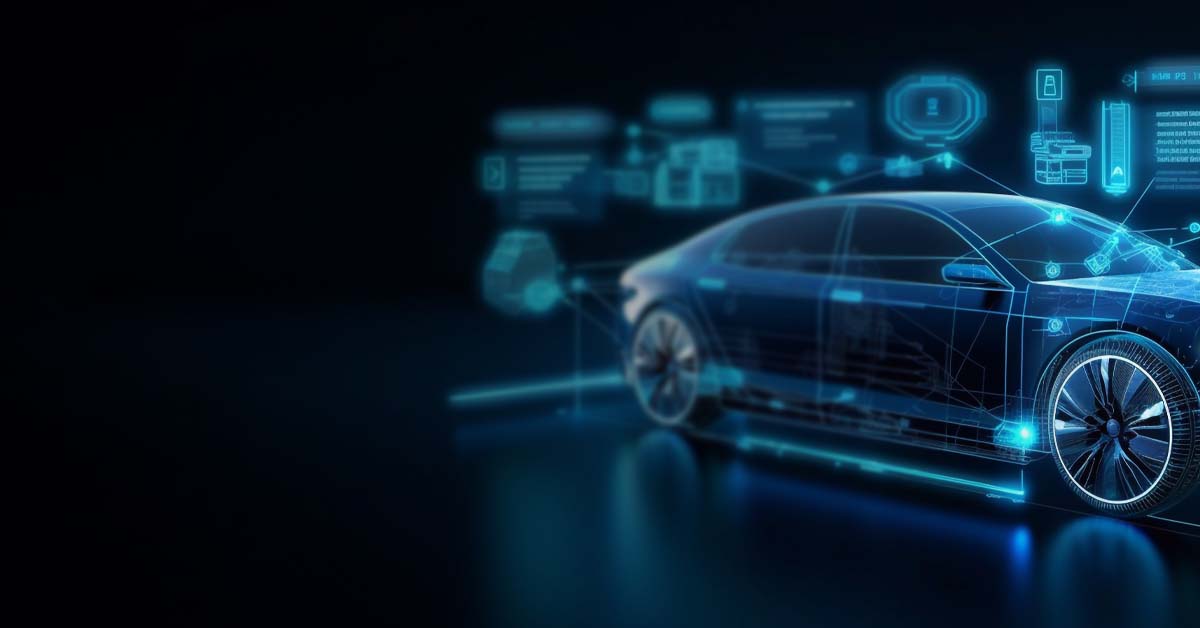
The 5 Benefits of AI-Driven Design in Engineering
AI can open up new opportunities for innovation and problem-solving that were previously unattainable, leading to an expansion of the engineering field rather than its contraction.
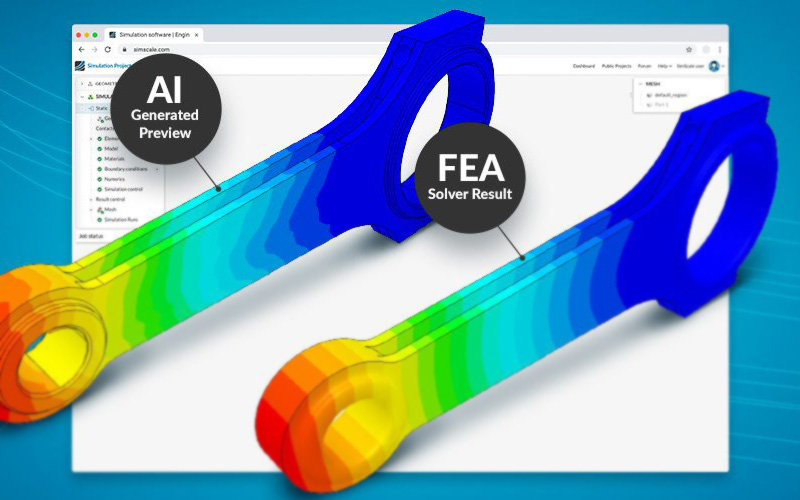
Real-Time Physics Predictions Empowered by AI
Upgrade your browsing with AI-generated physics predictions for real-time interactivity.
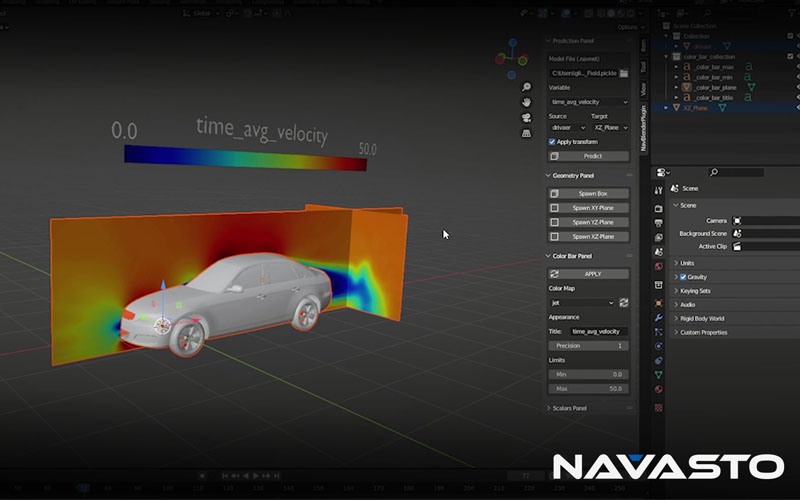
Enhancing Aerodynamics With Artificial Intelligence: A Revolution in Engineering
Learn how NAVASTO’s AI technology is revolutionizing product development & EV aerodynamics. Discover the power of real-time insights for engineering.
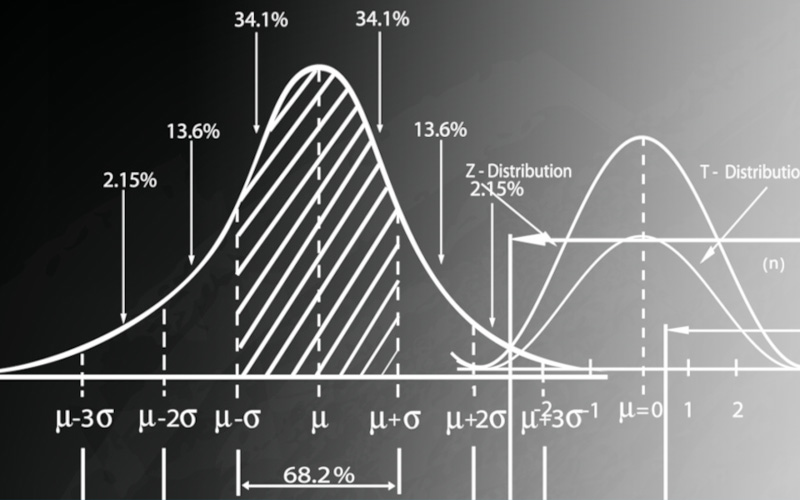
Probabilistic Gaussian Processes – An Approach To Stable Response Surface Modelling for Noisy Processes
Response surface models based on Gaussian Kernels, such as Kriging, require the estimation of hyperparameters to fit the model to the training data. Commonly, this estimation is made by exploiting the maximum marginal likelihood (MML) function. However, this is not an easy optimization problem due to the topology of the MML function. An alternative – and more robust – approach to hyperparameter estimation can be based on Bayesian statistics.
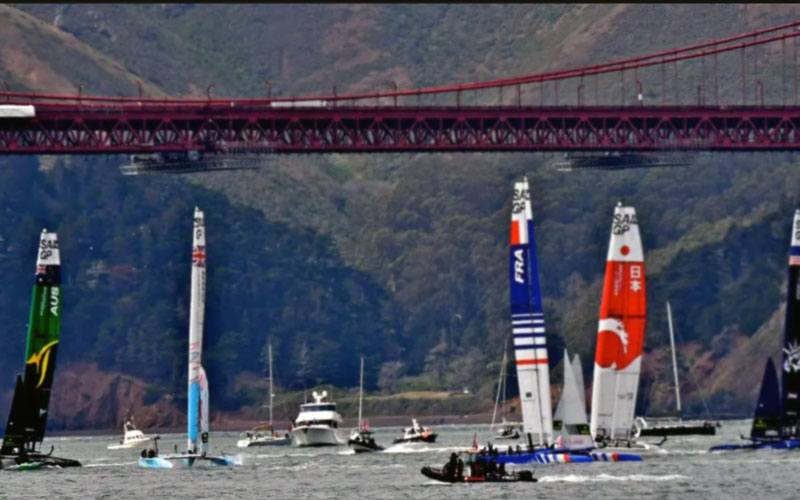
The Future of Maritime Travel: The Next Phase in Ship Design Evolution
Ship designers face challenges like emissions regulations, efficiency optimization, and future-proof propulsion. AI advancements enable real-time prediction of flow fields and scalar quantities, accelerating product development. A pilot boat project used parametric models and simulations with CAESES and Simcenter STAR-CCM+. Machine learning models based on geometric parameters facilitated rapid design processes. This blog discusses the project, including the creation and accuracy of the Reduced-Order Model (ROM).

Ready to explore how AI can fast-track your problem-solving?
Let's talk.